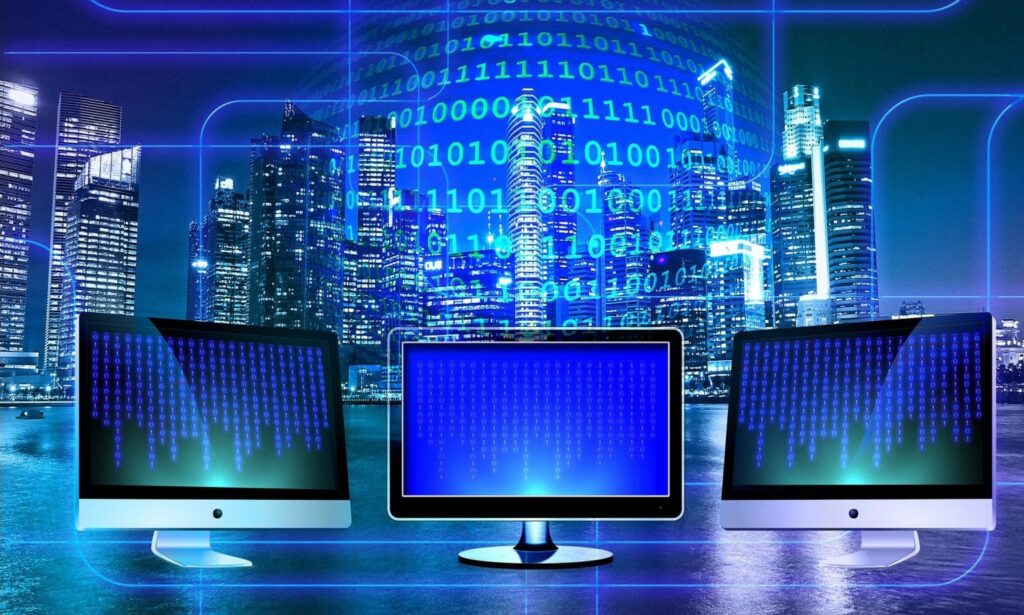
Which of the following most accurately describes data lifecycle management (DLM)? Data lifecycle management (DLM) refers to the systematic approach of managing the flow of an information system’s data from its inception to its eventual archiving or deletion. DLM involves various automated systems and policies to ensure efficient data handling through its lifecycle. For life sciences organizations, maintaining data integrity throughout each phase is crucial.
Core Objectives of Data Lifecycle Management
The main objectives of DLM are to maintain data security, integrity, and availability. This ensures that data is protected, accurate, and accessible when needed, despite being stored across diverse locations and platforms, such as on-premises, cloud, or hybrid environments. Establishing rigorous data integrity measures at every stage of the Data LifeCycle is imperative.
Key Goals of Effective Data Management
Experts outline three primary goals for a robust DLM strategy:
- Data Security and Confidentiality: Ensuring data is secure from unauthorized access and breaches.
- Data Integrity: Maintaining data accuracy and consistency across all storage locations. In the life sciences sector, the integrity of data must be preserved meticulously throughout its lifecycle.
- Data Availability: Ensuring data is accessible for authorized users without interruptions.
Phases of Data Lifecycle Management
DLM can be segmented into distinct phases, each critical for managing data throughout its lifecycle:
Phase | Description |
---|---|
Generation and Collection | Data is created by various sources and collected depending on its nature. This includes data acquisition, entry, and capture from devices used in various organizational processes. |
Storage and Management | Stored data is maintained to ensure its security, integrity, and protection. Implementing robust backup and recovery processes is essential for retaining data integrity during its lifecycle. |
Usage and Sharing | Data is utilized by authorized users to perform their operations efficiently. Maintaining an audit trail for critical data ensures that modifications are traceable. Data may also be shared outside the organization. |
Archiving | Data no longer actively needed is securely stored for long-term retention. A data archive is a place where data is stored without maintenance or general usage, available for restoration if needed. |
Destruction | Obsolete data is securely purged following data protection regulations. It is crucial to ensure that all copies of data are removed after exceeding their regulatory retention periods. |
Augmenting DLM with Technology
Modern DLM solutions incorporate AI and machine learning to enhance the automation of data management tasks, such as data tiering and compliance checks, demonstrating a shift towards more dynamic and responsive DLM systems.
Distinguishing DLM from ILM
While DLM focuses on managing data based on general attributes like type and age, Information Lifecycle Management (ILM) offers more granular control, crucial for compliance and specific data retrieval needs. The distinction is particularly important in fields like life sciences where data integrity and validation are critical.
Final Insights on Data Management
Whether through hierarchical storage management or more advanced ILM tools, the essence of data lifecycle management remains a comprehensive strategy to safeguard and optimize the use of data across its lifecycle. This article delineates the profound impact of DLM on organizational data management and its pivotal role in compliance and operational efficiency.